Automation bias may be the end of us all
We need new paradigms for thinking about a world where machines can teach themselves to do things humans wouldn’t.
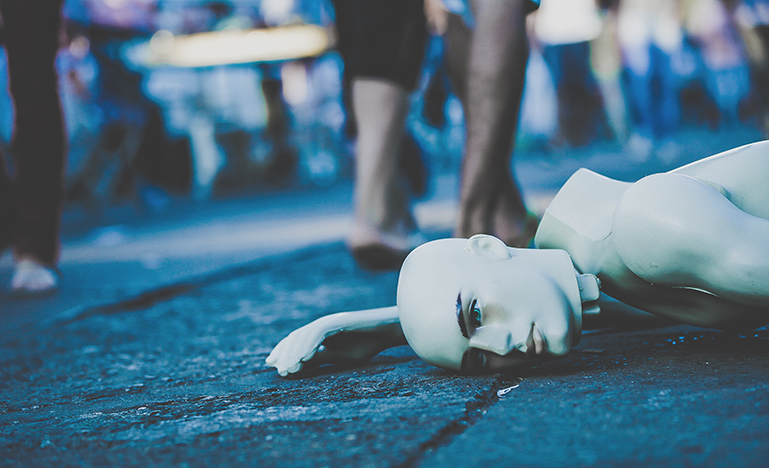
Game 2, Move 37. A machine, AlphaGo, made a move in the game of Go that no human would have made.
AlphaGo itself worked out the chances of a human making that move as one in 10,000.
That 2016 game marked a turning point in the field of artificial intelligence – the machine had been able to teach itself to make such a “genius” move.
Two games later, AlphaGo’s human opponent, Lee Sedol, one of the world’s best Go players, made a move that AlphaGo did not expect – another one in 10,000 move that threw AlphaGo off its game so badly that Sedol won his first and only game of the five-game match.
One thing that stands out for the world of AI in these games is that playing against the machine made the human player better – if AlphaGo hadn’t startled him so with Move 37, Sedol might not have made his own move, which followers dubbed “God’s Touch.”
The thing that’s important about Game 2, Move 37 as far as the law is concerned, says University of Ottawa professor Ian Kerr, is that the machine making an unprogrammed move was not a matter of product liability or failure – the move was unprogrammed but not, in a real sense unanticipated.
"We need new paradigms for thinking about a world where machines can teach themselves to do things humans wouldn’t..."
We need new paradigms for thinking about a world where machines can teach themselves to do things humans wouldn’t, Kerr told a recent CBA Privacy and Access to Information Law conference session on artificial intelligence.
He points out that the Ontario Electronic Commerce Act of 2000 says electronic agents can enter into contracts – but those agents might act on behalf of a principal who may not have authorized the transaction, but is nonetheless responsible for it.
“I think we’re at a point where we have to think of AI and robots as being at a point where they can formulate beliefs and opinions without human interaction. We should prepare for the future by understanding the near- and medium-term consequences of what’s before us.”
That said, he points out that it’s a “non-trivial” problem to get from pattern recognition, which is the general state of play right now when it comes to AI for legal purposes, to machine intelligence – and it’s a leap that has failed several times already.
The panel included Sylvia Kingsmill from KPMG, Chelsey Colbert from Fasken Martineau DuMoulin and moderator Sinziana Gutiu of Dolden Wallis Folick.
Colbert says the trend is toward either augmenting or replacing humans, and it’s something we’ll see even more of as we discover they are more efficient than humans and unbiased.
Kerr calls that automation bias – people placing too much trust in machines. “One thing to keep in mind is that you can’t assume that the system is accurate.”
Algorithms are unemotional and mathematical, but does that mean they’re unbiased?
How do you remove the bias from the humans when they’re teaching the machines, or from a machine when it’s teaching itself, in order to arrive at unbiased data, asks Kingsmill.
Algorithms are unemotional and mathematical, but does that mean they’re unbiased?
“For the output not to be biased, you need a lot of data, but how do you achieve data minimization when you have to collect so much data in order to be unbiased?”
When Gutiu asked how the market is responding to the privacy implications of AI and data collection, Kingsmill responded that every report on AI “has something to say about algorithm transparency, the need to respect privacy, and a new term that’s in vogue: ethics.”
Ethics informs the law, says Kingsmill, but you can’t regulate ethics. And while we’ve been talking a lot about the ethical use of data in Canada, the market never waits. Clients come wanting to launch a new product, and they’re ready to go.
So what, Gutiu asked, should people think about when a file with AI implications lands on their desk?
First of all, Colbert says, most people won’t realize the AI implications. But lawyers and ethicists should be involved at the design stage because until regulations are governing the sector, it will come down to corporate responsibility.
In the absence of regulations, says Kingsmill, a company has to ask itself whether it has values or principles that it can follow, and whether they can be operationalized. Look at the people around the table: your coder, the privacy agency, your lawyer, ethicists – do they all speak the same language? Do they have the same beliefs? You have to make sure they are all on the same page.
Delegating decision-making is the same whether you’re delegating to an employee or to a machine, says Kerr. You think about what tasks are appropriate to delegate instead of simply adopting some app because it’s popular. You should know what that app is and how it works, the same way that you would assess an employee’s ability and suitability to carry out a particular function.
While fear mongers would have us worry about the possibility of intelligent machines turning on humans, Kerr says for him the more dystopic vision of the future is where we’ve placed our confidence in them to such an extent that we’ve lost our own capacity to do the work, and then machines fail us.